Decentralizing AI: How Bagel sparks open innovation through community-driven platforms
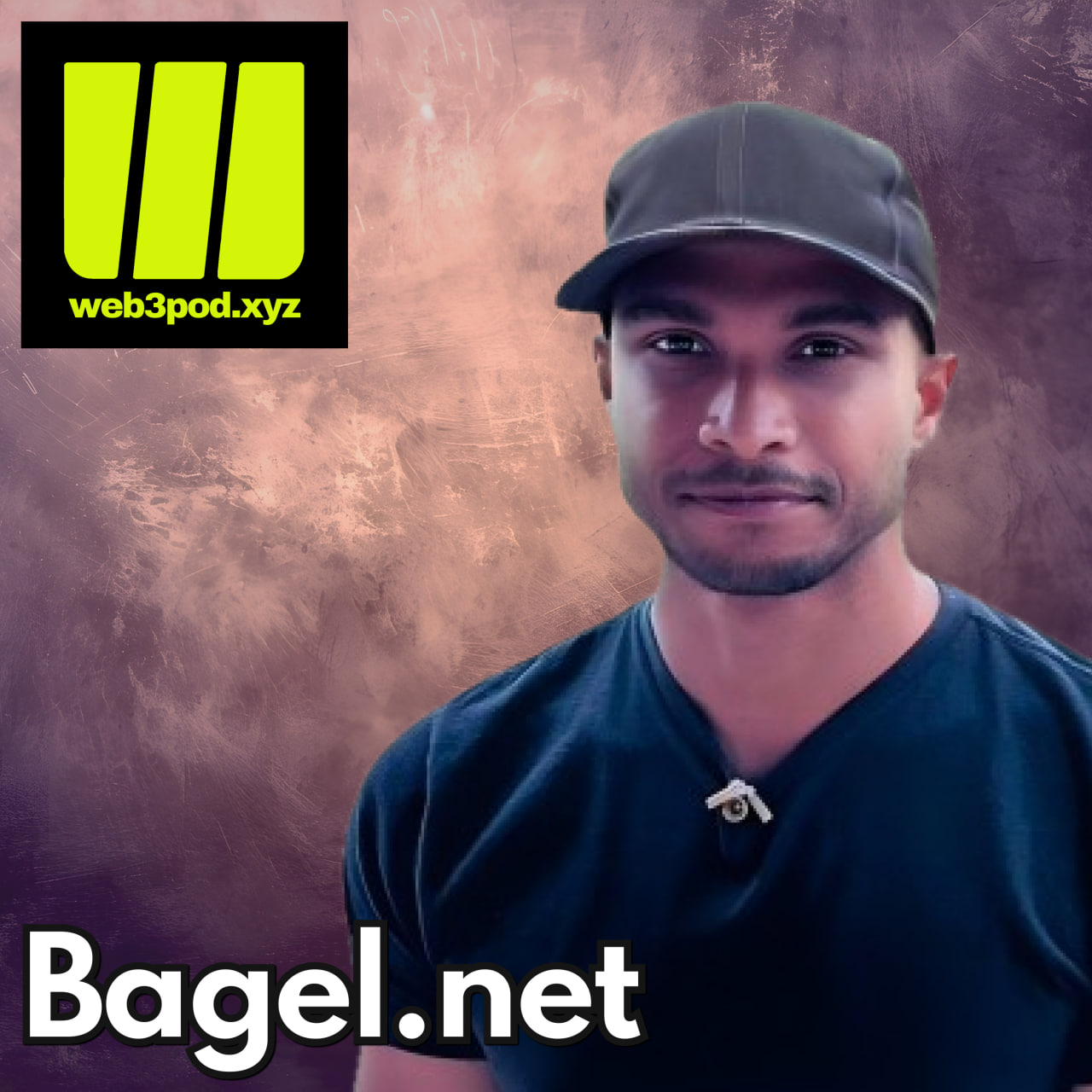
The inspiration behind Bagel
Shawn began by detailing his motivations for building a more transparent, community-oriented AI platform. Traditional AI development models often rely on large organizations hoarding data and controlling the flow of information. This model can discourage grassroots innovation, placing significant power in the hands of a few.
In response, Bagel is structured to empower smaller entities—startups, research groups, or even individual developers—to contribute AI modules and datasets that others can build upon. The goal is to create an interconnected network of solutions, where each participant benefits from collective knowledge while maintaining ownership of their own intellectual property.
Addressing the “black box” problem
AI’s complexity frequently leads to “black box” scenarios where it’s hard to understand how decisions are being made. Shawn argued that decentralized models encourage openness, allowing more people to scrutinize model architectures and data sources. An open ecosystem:
- Enhances trust: Users can see the sources of training data and how AI models arrive at results.
- Promotes accountability: Contributors have to stake their reputation or tokens, ensuring they remain responsible for the content and performance of their models.
- Drives innovation: When researchers and developers have the liberty to iterate on open models, they often produce creative, unanticipated solutions.
On Bagel’s platform, smart contracts serve to track contributions and usage. This structure can also automate revenue sharing, ensuring that those who improve a model or provide valuable datasets are rewarded fairly.
Incentivizing collaboration
A recurring theme in this episode was how Bagel designs incentives for contributors. Building robust AI models requires more than coding skills; it demands varied data, specialized analytics, and the capacity to identify practical use cases. Bagel’s approach allows participants to earn based on how much their additions, enhancements, or data sets are utilized.
The result could be a virtuous cycle: as more developers join, the pool of resources grows, attracting additional participants who see tangible rewards for their efforts. This decentralization not only disperses risk but also distributes the upside, making it more likely that genuinely useful solutions will emerge.
DeFi meets AI: Funding and governance
Shawn also touched on how decentralized finance (DeFi) can intersect with AI ecosystems. Funding for AI research is often scarce, especially for smaller players. Through token-based governance, communities can vote on which projects to fund, helping to channel resources into initiatives the community deems worthy. This democratic approach can unearth niche opportunities that might be overlooked by traditional venture capital.
Such governance models, however, aren’t immune to pitfalls. Large token holders could dominate votes, and complex proposals might overwhelm less technical users. Shawn advised maintaining a balanced governance structure, where experts and community stakeholders collaborate to set priorities. By combining transparent allocation of capital with open technical development, the AI-crypto synergy could reshape how we invest in AI research.
Real-world use cases
One of the highlights was hearing about use cases already surfacing:
- Collaborative data sets: Multiple entities pooling data for training algorithms that tackle real-world problems like climate modeling or genomic research.
- Shared AI modules: Developers building specialized modules—like natural language processing or image recognition—that others can integrate into their dApps.
- Localized AI solutions: A decentralized network can support niche languages or specialized industries often neglected by big tech firms.
Shawn pointed out that most breakthroughs in AI stem from broad, diverse inputs. By lowering the barriers to collaboration, Bagel hopes to foster projects that might not even be conceived within the confines of a single organization.
Balancing privacy and openness
Of course, the question of data privacy looms large. Many organizations can’t share sensitive data openly due to legal or competitive reasons. Bagel’s strategy is to enable differential privacy techniques and other cryptographic tools that let entities maintain confidentiality while still contributing to a shared model. This ensures that proprietary information isn’t exposed, but the community can collectively benefit from the results.
Shawn underscored that these features are still evolving. Decentralized AI platforms must demonstrate their trustworthiness, potentially through rigorous audits, bounties for security vulnerabilities, and ongoing community vetting. Maintaining a balance between privacy and collaboration is a delicate act, but crucial for long-term sustainability.
Bridging Web2 and Web3 AI
Many companies have already invested heavily in AI systems on centralized platforms. Shawn discussed a hybrid approach where traditional players can dip their toes into decentralized AI by exposing part of their data or model architecture in a controlled fashion. This incremental method could ease them into Web3’s principles of transparency and shared ownership without requiring a full overhaul of their current infrastructure.
Over time, if these companies see tangible benefits—like better model accuracy, cost savings on data acquisition, or stronger user trust—they might transition more of their AI stack onto decentralized frameworks. This is the type of step-by-step process that can build broader acceptance for AI’s evolution under a Web3 lens.
Challenges ahead
Despite promising opportunities, there are challenges to consider:
- Regulatory uncertainty: The legal landscape for token-based governance and decentralized data sharing is evolving.
- Market education: Potential collaborators must learn how blockchain-based incentives and data sharing really work.
- Technical scalability: AI workloads can be resource-intensive; existing blockchains may struggle to handle the computational demands without additional scaling solutions.
- User adoption: Attracting a critical mass of contributors is essential for any network effect, making marketing and community building crucial aspects.
Bagel’s team remains optimistic. They argue that collective intelligence, combined with robust cryptographic foundations, can outpace what any single organization could achieve alone.
Final thoughts
Shawn’s vision reimagines AI development as a community-driven enterprise, rather than a battleground of top tech firms. Bagel’s platform makes it possible to build, share, and refine AI solutions on a global, open network—with participants fairly rewarded for their contributions. This approach might pave the way for more ethical, innovative AI—a landscape where breakthroughs originate not just in elite labs, but from the grassroots.
If you’re passionate about AI’s future or simply curious how decentralization could accelerate machine learning breakthroughs, this episode offers an eye-opening perspective. It’s a testament to the belief that open collaboration and shared incentives can spark an era of invention that transcends corporate silos.
Listen to the full conversation
For a deeper look at Shawn’s background, Bagel’s technical foundations, and practical use cases, check out the entire episode here:
- Spotify: Listen here
- Apple Podcasts: Listen here
Feel free to share this episode with colleagues or friends who are exploring new paradigms for AI or simply want to understand what decentralized technology brings to the table.